IMoSA – Imitation Mechanisms and Motor Cognition for Social Embodied Agents
Amir Sadeghipour and Stefan KoppAbstract
This project explores how the brain's motor cognition mechanisms--in particular, overt and covert imitation or mental simulation--are employed in social interaction and can be modeled in artificial embodied agents. Taking a novel perspective on communicative gestures, we develop a hierarchical probabilistic model of motor knowledge that is used to generate behaviors and resonates when seeing them in others. This allows our virtual robot VINCE to learn, perceive and produce meaningful gestures incrementally and robustly in social interaction.
Research Questions and Methods
Neuroscience has shown that in humans the perception and production of movements is grounded in common sensorimotor structures. This affords core abilities of social interaction (coordination, imitation, mentalizing) and is also found when interacting with sufficiently humanoid artificial agents. The mechanisms, however, are not well understood nor utilized for obtaining similar abilities in embodied interactive systems. We try to capture and model these mechanisms to investigate natural and embodied social interaction with the virtual robot VINCE. Focusing on communicative hand gestures, motor cognition is defined to encompass all processes involved in the production and comprehension of one's own and others' gestures. We adopt the idea of shared motor structures that represent how own gestures are performed (generation) and are also exploited to make sense of the gestures other's are performing (perception), constituting a "perception-behavior expressway" (Dijksterhuis & Bargh 2001). Our research questions are: (1) How can such structures be modeled and linked into gesture perception and production processes? (2) How are they acquired and learned incrementally during social interaction? (3) How can these structures root in observed or performed instances of gestural behavior (exemplar-based approach), but eventually afford the generativity of human social behavior? (4) How can we, based on this model, simulate human social capabilities such as incremental anticipation, mutual coordination and alignment in artificial agents? Our technical testbed consists of marker-free body tracking and speech recognition technology, with which VINCE can engage with a human in verbal and nonverbal interaction. In this setup, VINCE is equipped with a computational model that puts motor knowledge in the middle between behavior generation and perception, both processes using and affecting these structures simultaneously (see Figure). In this way the agent perceives and recognizes gestures by comparing them to its own motor simulation, i.e. how it would perform that gesture. The model is graph-based and hierarchical, representing motor knowledge on three different levels of abstraction, from small movement segments (motor commands), to whole performances of a gesture (motor programs), to more general schema representations (motor schemas) leading into levels of social or communicative intention. The representation is defined as a Bayesian network. Updated probabilities of each node, resulting from Bayesian inference when predictions (forward models) are compared against actual observations, indicate neural activation of the corresponding sensorimotor element. Activations spread within each level and float in-between adjacent levels (bottom-up/top-down). Since they cease slowly, interleaved generation and perception — as in reciprocal interaction — come to affect each other.
Outcomes
The model has been implemented at the levels of motor commands, motor programs and (to some extent) motor schemas. It has been tested against real-word hand-arm gesture data in an imitation game scenario with the virtual robot VINCE. The hierarchical structure of the motor knowledge together with its role as link between behavior perception and generation are found to support the basic processing of social behavior and, additionally, core mechanisms of social interaction, from alignment and mimicry to imitation learning and understanding behaviors through embodied simulation. Bottom-up processing realizes fast, incremental behavior recognition and interpretation; top-down flow of activation realizes behavior generation and simulate attentional and perceptual biases during perception. Overall, this approach takes a novel, integrated perspective toward gesture processing in an embodied manner. See publications for results on the performance of the model during social interaction, in terms of recognition, imitation learning and alignment; see an older version of the interaction scenario in the video.
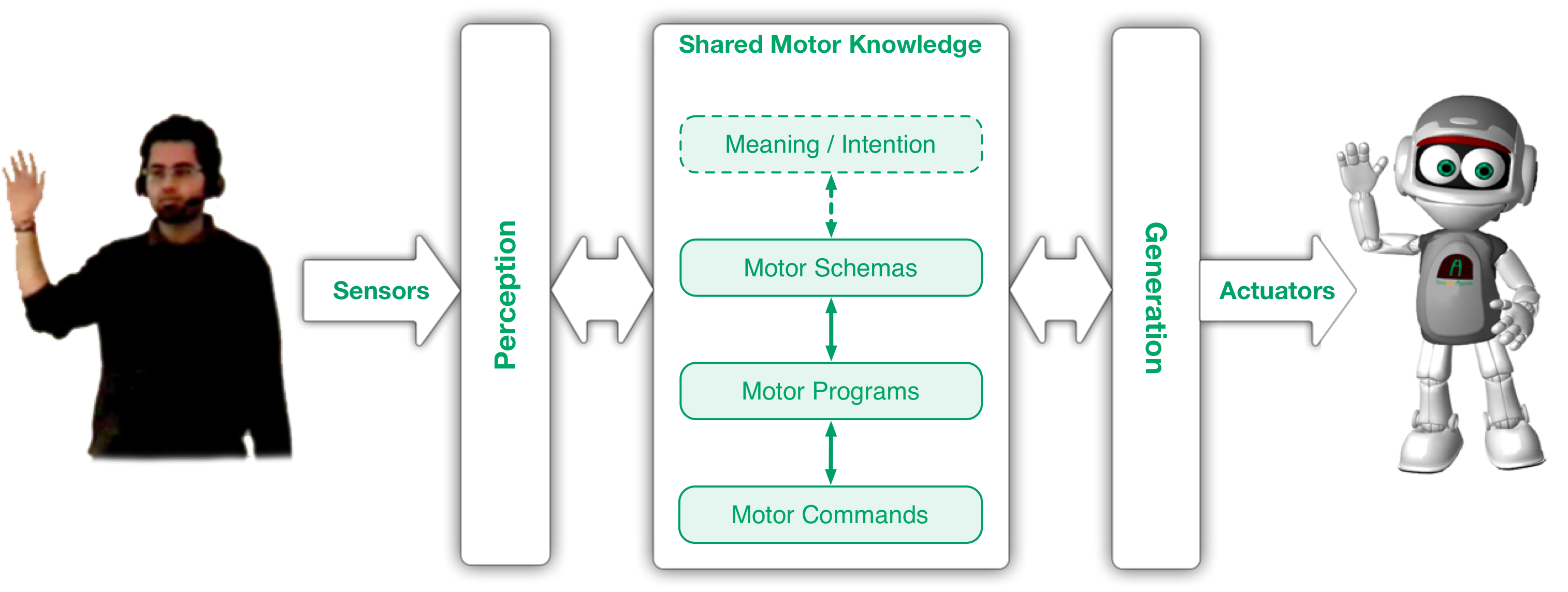
The overall model for embodied gesture perception and production grounded in a shared motor knowledge.
Video
Publications
-
A. Sadeghipour, R. Yaghoubzadeh, A. Rüter, and S. Kopp. Social motorics - towards an embodied basis of social human-robot interaction. Human Centered Robot Systems, pages 193-203, 2009.
[PDF Fulltext] [BibTex Cite] [DOI URL][Wordle]
-
S. Kopp, K. Bergmann, H. Buschmeier, and A. Sadeghipour. Requirements and building blocks for sociable embodied agents. KI 2009: Advances in Artificial Intelligence, pages 508-515, 2009.
[PDF Fulltext] [BibTex Cite] [DOI URL][Wordle]
-
A. Sadeghipour and S. Kopp. A probabilistic model of motor resonance for embodied gesture perception. In IVA '09: Proceedings of the 9th International Conference on Intelligent Virtual Agents, pages 90-103, Berlin, Heidelberg, 2009. Springer-Verlag.
[PDF Fulltext] [BibTex Cite] [DOI URL][Wordle]
-
A. Sadeghipour and S. Kopp. Embodied Gesture Processing: Motor-based Perception-Action Integration in Social Artificial Agents Cognitive Computation, pages 1-17, New York, 2010. Springer.
[PDF Fulltext] [BibTex Cite -> see URL] [DOI URL][Wordle]
last update: 2011-6